501c3, meet GPT3
This week’s edition of The Process is meant to introduce my friends from area code 501c3 ❤️🌎 what’s happening in area code GPT3 🤖. Most…
This week’s edition of The Process is meant to introduce my friends from area code 501c3 ❤️🌎 what’s happening in area code GPT3 🤖. Most of my career has been in the nonprofit sector and now, with two years of experience as a tech founder, I’m sharing my learnings from where these two worlds meet.
This piece provides a round-up of core concepts that help to explain how AI works, common phrases we’re likely to hear, and key organizations shaping the sector. It sets a foundation I’ll reference in future pieces about the implications of technology, especially AI, on the nonprofit and philanthropic sector.
What is artificial intelligence?
In simple terms, artificial intelligence is the ability of computer systems to perform tasks that normally require human intelligence to do. Humans have been replacing our own physical labor with machine labor for thousands of years. For the last several decades this trend continued as we replaced mental labor with software like spreadsheets and simple automations. With AI, we’ve reached the point that our tools are capable of replacing some creative labor, as well.
A defining characteristic of AI is its ability to improve its performance by learning. This is in contrast to other forms of software which must receive explicit instructions from programmers to change performance.
How does AI work?
Unlike traditional software, programmers are not writing code explicitly directing the system to perform specific actions. Instead, AI systems are programmed to analyze huge sets of data to look for patterns and relationships within that data.
For example, to train AI to recognize images of dogs, a system would be given a large set of images containing dogs and told these pictures contain dogs. Then it would be given another large set of images without dogs and told these are pictures of things other than dogs. The AI would discover patterns common to the pictures with dogs and which are not present in the pictures without dogs. With enough training, the system can use these patterns to effectively recognize and even generate new images of dogs.
What are neural networks? What is deep learning?
The way this kind learning ability is achieved is by training intricate computer systems called neural networks, which are inspired by the complexity and architecture of our own brains. Data fed into AI neural networks flows through layers of analysis comprised of millions of what can be thought of as sensors. Each sensor is narrowly focused on a particular aspect of the incoming data. Each sensor records readings and relationships with other sensors and over many layers of analysis and huge amounts of data, patterns emerge across millions of data points that have been created. This process is called deep learning because of the depth created by the many layers of analysis.
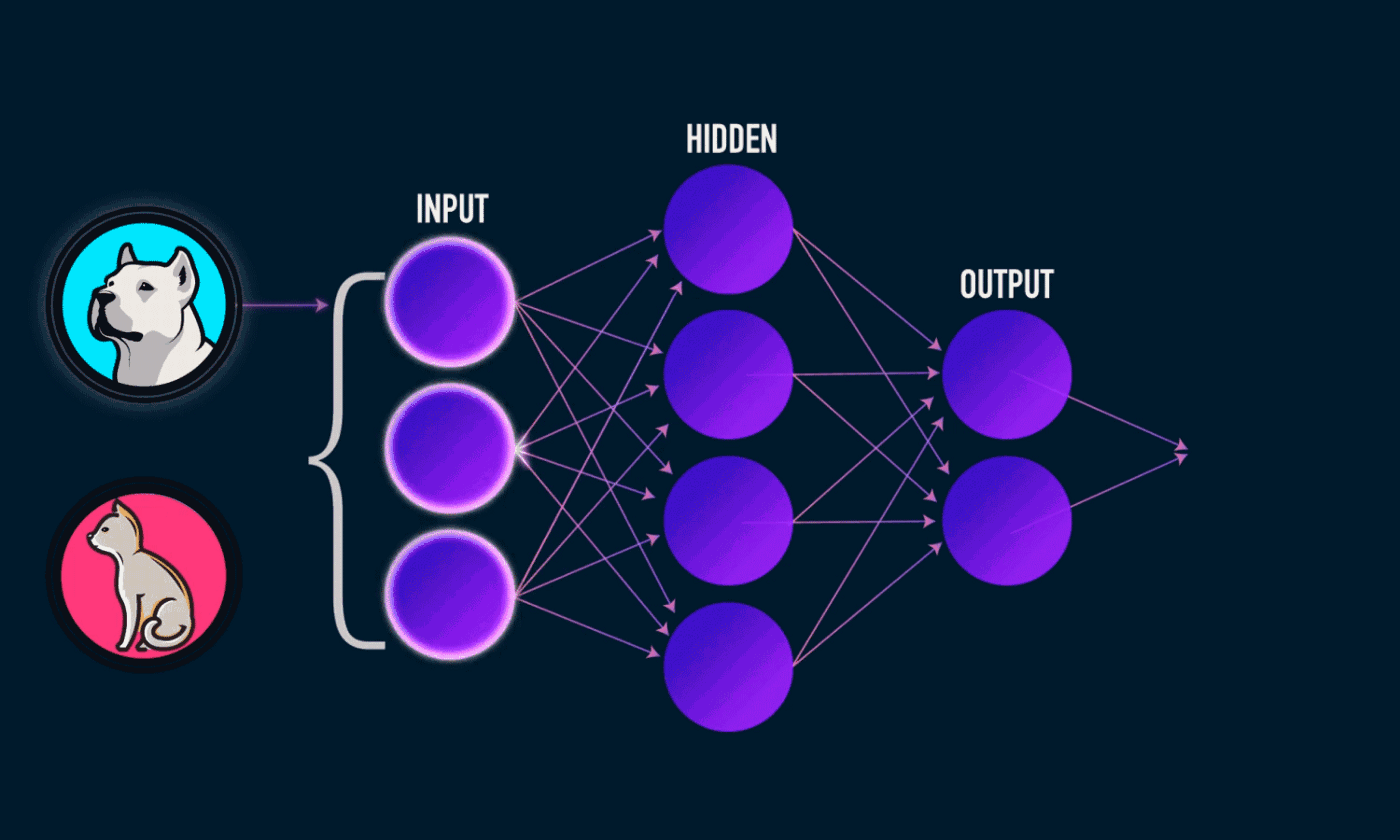
How does AI generate text and images?
Once deep learning systems have been trained, once they ‘see’ patterns, they develop a prediction ability. Take a look at the following example from ChatGPT:
My prompt didn’t contain any instructions, context or clues about what that famous line is from, yet ChatGPT was able to accurately finish the nursery rhyme. The dataset it was trained on likely contained numerous instances in which “Mary had a little lamb” is followed by “Its fleece was white as snow.” Based on my prompt, ChatGPT determined based on pattern recognition, the most likely words to follow and respond with.
What if AI makes a mistake?
A very big vulnerability of such a system is it does not have the ability to consider the truthfulness or accuracy of the content it generates. Social media has been full of screenshots from people who have been posting erroneous responses from ChatGPT, such as the following.
Both Nicaragua and Honduras have larger land areas than Guatemala. In this case, I imagine since Guatemala has the largest population of any country in Central America (excluding Mexico), there may have been numerous instances in ChatGPT’s training data in which Guatemala is referenced as the “largest country in Central America”, wherein the writing is referring to its population, rather than land mass. Because ChatGPT is using probability to generate the response it creates, the accuracy of the outputs is dependent on the rate of information in its training data that looks like the prompt it has been given. I expect accuracy and new solutions to verify factual information to improve rapidly, but for now it’s important to double check anything you’re getting from AI sources.
To review, deep learning systems take in huge amounts of training data, which is passed through neural networks, which take readings and record relationships within the data to reveal patterns across millions of data points. These patterns allow predictive algorithms to generate all kinds of content by constructing it, piece by piece, based on statistical likelihood. A deep learning system trained on enough pictures of dogs can learn patterns and relationships common to dog images and create new pictures of dogs based on probability.
Who is building AI systems and companies?
Building these sophisticated AI systems is an extremely complicated and expensive process, which means the world’s largest and most well-resourced technology companies are in the best position to do so. Leading American companies working on AI include Alphabet Inc. (Google), Microsoft, Amazon, Apple, Meta (Facebook), IBM, and OpenAI. Most experts believe only a handful of highly capable AI systems will emerge worldwide, upon which other companies, like my own, will build layers of refinement and specialized interfaces to allow people to use AI-powered tools.
The company making the most news at the moment is OpenAI, the AI research company behind ChatGPT, a sophisticated chatbot millions of people have been interacting with online. Those three letters, GPT, stand for Generative Pre-trained Transformer, which is a type of neural network architecture. The word ‘generative’ references the ability to create novel outputs, the word ‘pre-trained’ speaks to the process of feeding these networks large amounts of data from which their patterning and predictive abilities arise, and the word ‘transformer’ relates to the way the meaning of inputs is determined.
After the release of OpenAI’s ChatGPT in late 2022, Google’s leadership immediately declared an internal ‘code red’ emergency, calling the company to respond to what they perceive as a potentially existential threat to their dominant search and advertising business. That Google, a company whose name has become a verb meaning ‘to search for things online’, feels such a level of concern about ChatGPT should be a signal to the rest of us about the tectonic shifts taking place at the bedrock layers of technology.
In 2019, Microsoft made a $1B investment in OpenAI and there are plans in the works to make another $10B investment. Microsoft is already incorporating aspects of ChatGPT into its own Bing search engine and its flagship office software.
Why is AI taking off now?
AI is not new, it comes from a lineage of research and development that is more than 60 years old. What’s new is AI doing things regular people pay attention to and care about, like generating images and text, and doing it at a level comparable to what humans are capable of.
The speed of AI’s progress is also influenced by massive financial investment in its development, availability of huge amounts of computing power, and massive quantities of online data and content, which is used to train AI systems.
We are likely to see an acceleration across all these factors including increased investment in AI, more computational resources allocated to AI development, and an explosion of AI-created content, which will provide even more data for training intelligent systems.
Glossary of AI terminology
I asked ChatGPT to help me write a glossary of some of the terms we’ve covered and the results were impressive. ChatGPT has likely been trained on huge amounts of factually consistent writing on the topic of AI, which gives me confidence its responses will be highly accurate, and I can easily verify them.
Here’s what it wrote:
Artificial intelligence (AI): A field of computer science that focuses on creating intelligent machines that can think and act like humans.
Machine learning (ML): A subset of AI that involves training algorithms to make predictions or decisions based on data.
Neural network: A type of machine learning algorithm modeled after the structure and function of the human brain.
Deep learning: A subfield of machine learning that involves using neural networks with many layers, which are able to learn and make decisions on their own.
Generative pre-trained transformer (GPT): A type of neural network architecture used for natural language processing tasks such as language translation, text summarization, and text completion.
You’re ready for trivia at nonprofit happy hour
Ok, nonprofit friends, you’ve got real intelligence about the artificial kind, and you’re ready to win trivia at the next nonprofit happy hour with your team! When the Quiz Master asks what GPT stands for, you’ll be ready. And if they ask about the implications of AI for the sector at large, tell them to subscribe to The Process because I’ll be writing a lot more about these topics and more.
Thanks for reading this first edition of The Process. While writing this post, I got feedback to do another post on the implications of AI on grant writing, in particular, so look out for that article in the weeks to come. Please feel free to share comments or you can email me at philip.deng@grantable.co
Philip Deng is the CEO of Grantable, a company building AI-powered grant writing software to empower mission-drive.